Talking Health Tech: How AI is Transforming Acute Care Documentation
Peter Birch, CEO of Talking Health Tech, interviews Justin Mardjuki at HLTH 2024

The following is a condensed summary of the interview available on Talking Health Tech. Remarks have been edited for clarity.
Peter Birch (CEO Talking Health Tech): We're all excited here at HLTH around the potential for AI to help improve efficiency and potentially quality of care. And an area that seems ready for immediate opportunity is documentation. Why is it specifically that the acute side requires that particular focus? I might look to think, well, all documentation requirements are the same.
Justin Mardjuki (CEO Sayvant): In 2023, CMS changed their billing guidelines for acute care to really shift away from listing the procedures and tasks that were accomplished in that encounter to focus much more on the medical decision making for that patient. As a doctor, you now have to sit down and write out your medical decision making - why you diagnosed a patient a certain way, why you dispositioned them in a certain way, and all the differential diagnoses that you rolled out.
As a clinician, that's why you went to school for 10 years. That’s like us having to explain why and how we do long division. So there's a huge potential for AI to take the decision and the diagnosis that the doctor's already made, and help explain their thinking based on all of the clinical and billing guidelines.
Peter Birch: How do you approach the issue of CIO and CTO looking at inputs trying to save time, improve efficiency, do more with less? Particularly in the acute care side, you know, quality and accuracy of information is paramount. We're in health care. We can't make any mistakes. What kind of safeguards are in place? How do you approach the need for innovating quickly, but doing things safely?
Justin Mardjuki: First, the traditional metrics around efficiency are the ones that ultimately matter. Medical groups are very good at looking at clinician efficiency, charges per hour, charges per encounter, right, and understanding how those trend up or down over time. And so the first most important thing is, are you at minimum not making an impact on those numbers and still be able to save physicians' time at the end of the shift? You can't get so enamored with a science project that it's not actually translating to real and clinical and efficiency results.
Second, on a much more granular level is thinking about those exact LLM AI guardrails. You can imagine thousands of tests that you run iteratively every time you change a prompt, every time you change an agent to make sure that it's only capturing procedures that were mentioned in the room or changing a doctor’s disposition. We put in pretty robust guardrails so you can track this actually on an agent by agent, encounter by encounter basis, and actively review them to have human quality review of these charts.
Peter Birch: I'm interested in also understanding your take on how these tools sometimes are like shadow IT. They're brought up through by clinicians because they see the benefit of using them first hand. Other times, as we're very good at doing in health care, enforcing technology down on the systems, e.g. ‘this is what you’re going to use now’. How have you found the best option for your particular tools within health care? Is it bottom up, top down, or a little bit of both?
Justin Mardjuki: I'm a bit biased because I grew up at the beginning of my SaaS journey at Box, which at the time was competing with Dropbox, and they took polar opposite approaches. Box was very enterprise IT driven, very compliance, security heavy. Dropbox was ‘use what you will, and we hope IT won't find out about it’.
And so in the AI Scribe space, we see a lot of solutions that are bottoms up clinician by clinician, put a credit card down, shadow IT. There's really an upper limit to what you could do with those kinds of solutions because they're not going to be aligned with how the group thinks about billing or quality measures. We've taken not necessarily a top down approach, but a more methodical approach where either at a site level or at a system level, you're able to enforce those kinds of guidelines across your physicians. And, the physicians also like it because it means less documentation burden for them. Architecting it in that way, I think is the right partnership between group leadership and clinicians on the ground.
Peter Birch: The last thing you want is for a clinician to be comfortable with the tool that they've brought in and then to later find out that it's, you know, not fine and it's been walked out. And so you wanna be able to ensure that stakeholders are aligned there. You and I were up on a stage yesterday, and I was moderating a conversation with you and Nika Duan about taking AI from 0 to 1 and engaging with the investment community as well as the providers too. What did you take away from those sessions and the discussions that we had there?
Justin: You can't walk 3 feet here without seeing AI and Large Language Models everywhere. There's a lot of talk of technologies without actual business problems behind them. We don't talk about ‘the cloud’ the way people might have 10 or 15 years ago. That's something that we need to remind ourselves of in health care. Ultimately, technology is in service of a real problem for an end user, and in my case, a clinician. And it doesn't matter if it's a large language model, small language model, AI, machine learning, or regression model.
Peter Birch: There's lots going on, lots of inspiration here at HLTH. What's really your focus over the next 6, 12, 24 months?
Justin Mardjuki: I'm really excited to see how Sayvant impacts the efficiency metrics we talked about. You're also seeing much more eagerness to partner across the ecosystem – you’re seeing RCM trying to get upstream into workflow. You're starting to see companies that own clinical content or clinical guidelines, want to embed in workflow. So that’s going to be a really interesting space over the next 6 to 12 months to think about how we incorporate these upstream or downstream partners while we have the clinician’s attention and focus at the point of diagnosis and assessment.
Related post
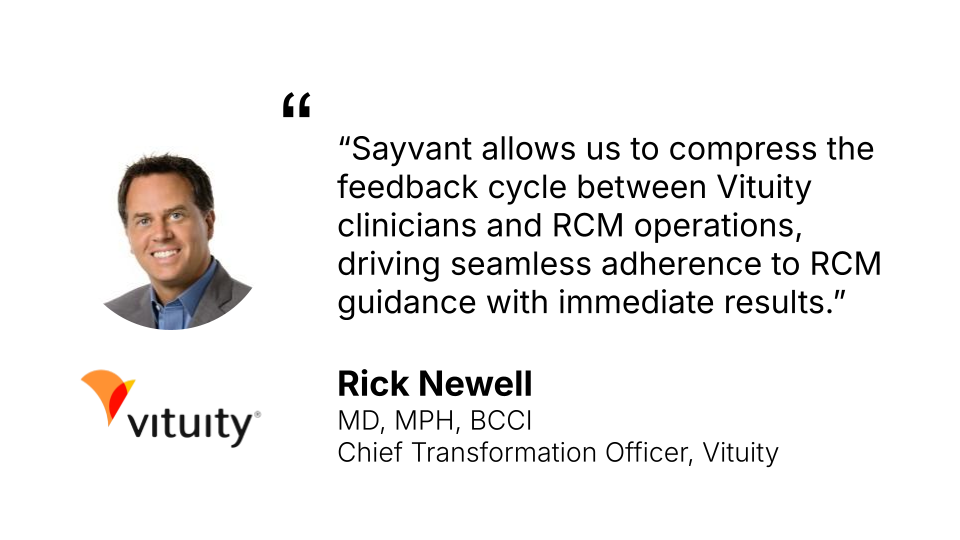
Sayvant Launches Real-Time RCM and CDI Integration for Enhanced Acute Care Charge Capture and Documentation Quality
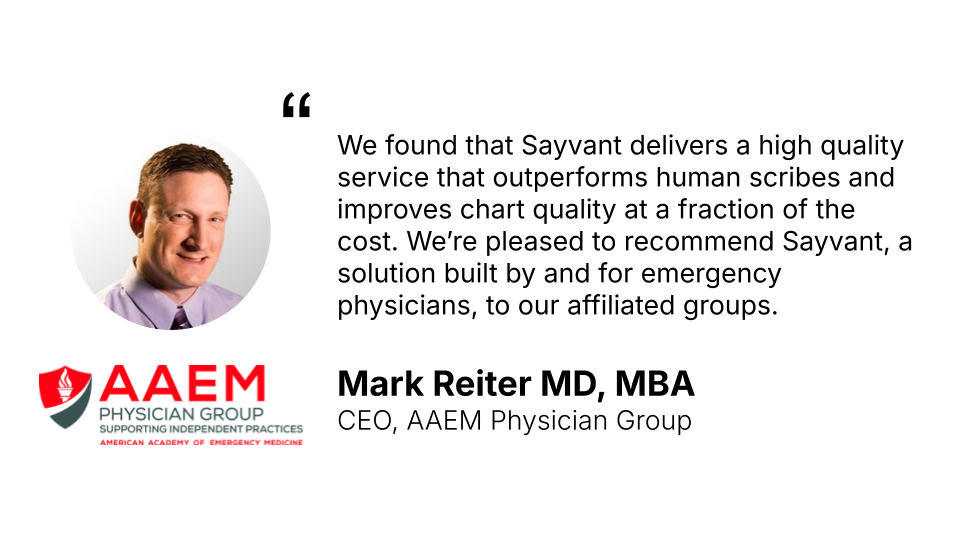